3 frontiers of AI technology set to raise the bar in PIM
May 28, 2024Viktor Bergqvist, Head of inriver’s Innovation Lab, outlines three rapidly evolving areas of AI and how they could take PIM to the next level.
Whether you’re an international brand or a small retailer, if you have a Product Information Management (PIM) system, you’re sitting on a data goldmine.
With a wealth of structured data at your fingertips that both humans and machines can interpret, there is no limit to the dynamic product stories you can tell or the insights you can glean.
Extracting the gold from this mine and turning it into a strategic asset across all B2C and B2B endpoints is getting easier all the time thanks to rapid advancements in large language models (LLMs). These artificial intelligence (AI) programs are trained on thousands if not millions of gigabytes of text from the internet. Here at the inriver Innovation Lab, we are on a mission to keep pace with the robots, exploring how to integrate their deep learning capabilities into our evolving PIM platform.
Here are three boundaries of machine learning we are pushing in 2024 to make the product data journey even easier and more impactful for our customers.
1. Multimodal content
Imagine you had to watch hours of product demo videos, analyze hundreds of photos, listen to multiple audio recordings, and wade through reams of customer reviews just to write a single product description for an exercise bike. Then imagine tailoring it for every consumer who might read it, from a new dad in Australia to a CEO in Brazil to a student in Latvia – in Latvian, of course.
You could do it. But even on the strongest coffee, it would take days if not weeks. And yet enrichment that drew on all that rich context would create very powerful product stories.
The inriver AI-powered Inspire module, launched in 2023, already integrates the power of OpenAI’s ChatGPT generative AI tool to automate content creation. You can generate hyper-localized and SEO-optimized product descriptions and social posts in multiple languages at the touch of a button. Our new Batch Editing function fast-tracks this process further by allowing you to generate descriptions for several products at a time, with preview windows to ensure you can validate the information before distributing it across all your digital touchpoints.
Thanks to advances in LLMs, our next big iteration of Inspire is likely to have ‘multimodal’ functionality, allowing users to input large amounts of imagery, audio, and even video to generate engaging product stories automatically.
OpenAI recently released its multimodal LLM ChatGPT-4o, which boasts several improvements over its predecessors – including the ability to accept any combination of text, audio, image, and video as inputs and generate any combination of text, audio, and image as outputs. While still strong in English, it also shows significant improvements in understanding and responding to text in other languages. Already talk has turned to GPT-5, slated for release in Summer 2024, and likely to be capable of generating even more nuanced and insightful content across different media formats and languages.
Meanwhile, Google has recently introduced its next-generation Gemini LLM, 1.5 Pro, which can analyze and process up to one million multimodal ‘tokens’ (basic units of data such as words) in one go – equivalent to one hour of video, 11 hours of audio, 30,000 lines of code, or over 700,000 words. That’s a significant jump in processing power from previous models.
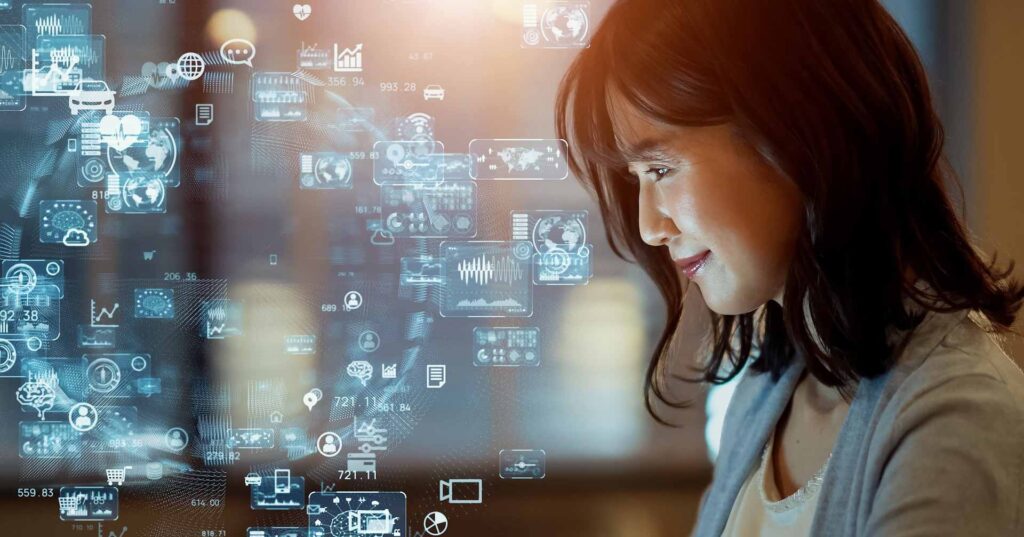
2. Efficient and affordable customization
Of course, the impressive capabilities of the major LLMs come at a cost.
Another interesting frontier of research for us is the smaller open-source LLMs appearing on the scene, such as Mistral and Llama. These don’t require as much graphics processing unit (GPU) to run and require much less processing power, which could make them a more efficient, agile, and cost-effective solution for some applications.
Their lower power consumption translates to a smaller environmental footprint – an essential consideration for all businesses. And their open-source nature allows developers like us to tinker with the code and customize it. This means we could potentially train the models for highly specialized PIM tasks and domains, leading to greater accuracy overall.
As these types of small-scale models rapidly evolve, we are looking at how we can leverage them inside the inriver PIM, perhaps even combining multiple smaller models to rival the performance of larger LLMs.
3. ‘Chain of thought’ prompting
Not so long ago, we all thought prompt engineering would become the next must-have skill for anyone working with AI. As anyone in the PIM space will tell you, a well-designed prompt can significantly improve the quality and accuracy of a product description. However, this requires an understanding of an LLM’s capabilities, the desired outcome, and the nuances of language. For a while, we thought that highly trained humans would play the clinching role here, not only previewing and validating content information but guiding the LLMs to generate compelling content.
Already, however, libraries are emerging that analyze your data and craft prompts for you, taking the mental legwork out of the equation. This is a key area of focus for us at the inriver Innovation Lab.
In earlier iterations of the inriver PIM, we provided a range of pre-configured standard prompts to help people get up to speed with AI technology. More recently, though, we’ve launched our Custom Prompt Editor, a customizable feature that lets you fine-tune prompts to the specific needs of your business. It means you can consistently produce on-brand product stories that resonate with your target audiences while speeding up the content validation process.
A promising area of development on the horizon is ‘chain of thought’ prompting. This technique is used in natural language processing to improve the performance and transparency of language models and involves guiding the model to generate a step-by-step explanation of its reasoning process as it works towards an answer. Instead of providing a direct response, the model articulates its thought process, effectively “thinking out loud.”
In the world of PIM, this could be helpful for understanding how the LLM arrived at a particular recommendation or comparison between products. Any discrepancies or biases could be picked up even more quickly, enabling iterative improvements that continually improve the accuracy of the LLM in PIM tasks. In today’s litigious and fast-changing regulatory climate, enhanced transparency could make chain of thought prompting a valuable tool for companies.
Machine learning: A sprint and a marathon for inriver
Barely a week goes by without an announcement of another AI breakthrough that opens doors to applications we never thought possible.
At inriver, we’re committed to staying plugged into the latest LLM advancements over the long term, tenaciously building and testing new ways to integrate them into our PIM platform, and then iterating at speed. By leveraging their superpowers, we can help our customers transform their data goldmines into complete, compelling, and compliant product information that maximizes profitability at every touchpoint.
want to see the inriver PIM in action?
Schedule a personalized, guided demo with an inriver expert today to see how the inriver PIM can get more value from your product information.
you may also like…
author
Viktor Bergqvist
Head of Innovation Lab
Viktor Bergqvist is a manager at inriver Innovation Lab, overseeing a variety of AI-powered innovations for the inriver platform.
read more